How Google uses AI to save Millions of Dollars on Computing Chip Design [Technique Tuesdays]
Improving Computing Chip Design with Reinforcement Learning
Hey, it’s your favorite cult leader here 🦹♂️🦹♂️
On Tuesdays, I will cover problem-solving techniques that show up in software engineering, computer science, and Leetcode Style Questions📚📚📝📝.
I put a lot of effort into creating work that is informative, useful, and independent from undue influence. If you’d like to support my writing, please consider becoming a paid subscriber to this newsletter. Doing so helps me put more effort into writing/research, reach more people, and supports my crippling chocolate milk addiction. Help me democratize the most important ideas in AI Research and Engineering to over 100K readers weekly. You can use the following for an email template.
PS- We follow a “pay what you can” model, which allows you to support within your means, and support my mission of providing high-quality technical education to everyone for less than the price of a cup of coffee. Check out this post for more details and to find a plan that works for you.
Following is an excerpt from my article- "AI x Computing Chips: How to Use Artificial Intelligence to Design Better Chips", a breakdown of Google's amazing open source AlphaChip. The original article covers many topics such as-
A history of AI in Chip Design.
Why older methods struggled with Chip Design.
Why Deep Reinforcement Learning is Promising for Chip Design
The core technical innovations that enabled AlphaChip
And a lot more.
The following excerpt focuses on point 3, breaking down the different techniques used to improve performance. In my opinion, AlphaChip is worth studying given the very promising economics-
Assuming AlphaChip reduces chip design time from 6 months to 1 week, and considering an average chip design team of 50 engineers with an annual salary of $150,000 each:
Potential savings per chip design: (150,000*50*(6/12–1/52))≈$ 3,605,769 (3.6 Million USD).
On top of this, if AlphaChip designs are 20% more power-efficient, this could translate to hundreds of millions of dollars saved for large power centers.
This makes it worth studying.
Executive Highlights (TL;DR of the article)
Starting from a blank grid, AlphaChip places one circuit component at a time until it’s done placing all the components. It’s rewarded based on the quality of the final layout. AlphaChip utilizes a new type of graph neural network that focuses on the connections between chip components. This allows it to understand how different parts of a chip interact and apply that knowledge to new designs, effectively learning and improving with each chip layout it creates.
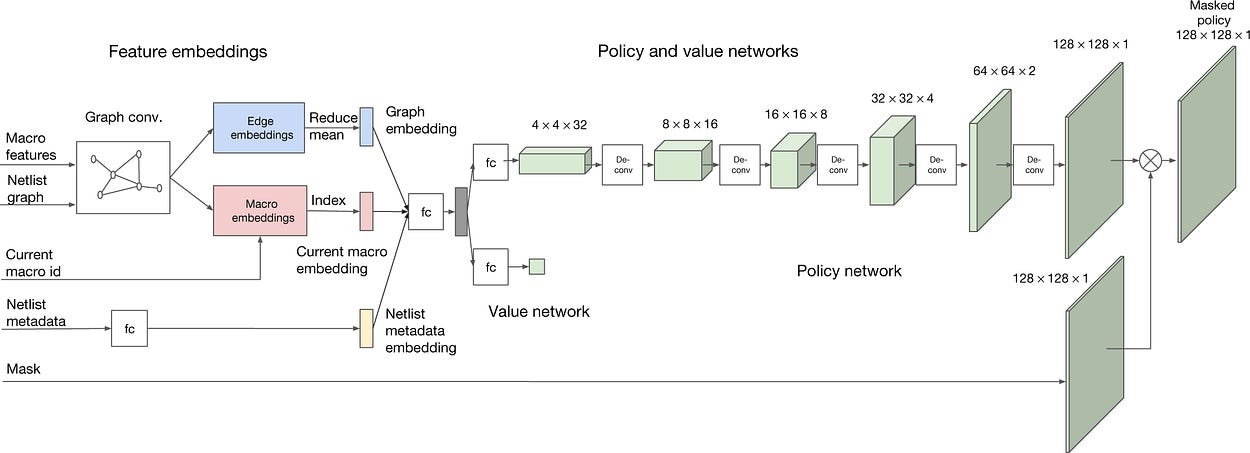
This relies on several components-
Edge-Based Graph Neural Network (Edge-GNN): Instead of just looking at individual components, this network focuses on relationships between components. This is crucial because component interactions (like wire lengths and signal timing) determine placement quality. Interestingly, the Edge-GNN is used not for predictions but as an encoder to create feature embeddings for the network prediction (shown in the image above). Seeing the focus on embeddings makes me very very happy.
Hierarchical Placement: Places large components (macros) first using RL, then handles smaller components with faster methods, making the problem tractable and improving our control on the outputs (it’s easier to control non Deep Learning methods).

Transfer Learning: AC pre-trains on multiple chip designs to learn general placement principles, like keeping frequently communicating components close together. We can use the base-level pretraining or fine-tune further to optimize gains for specific setups. Based on the results below- zero-shot seems like it does a pretty good job- which is a good indication that the representations are robust.
Custom Reward Function: Balances multiple objectives (power, timing, area) while enforcing manufacturing constraints.
RL Training: Uses Proximal Policy Optimization (PPO) to effectively navigate the enormous state space and learn stable placement strategies.
Aside from this, there are following are the other aspects of this setup that I thought were interesting.
Other Highlights
Constraint Handling: Embeds constraints by masking out illegal placements, allowing the agent only to consider valid actions during training, which improves training efficiency.
The Computational Efficiency: “In terms of resource usage, for pre-training we used the same number of workers as blocks in the training dataset (for example, for the largest training set with 20 blocks, we pre-trained with 20 workers) and the pre-training runtime was 48 h. To generate the fine-tuning results in Table 1, our method ran on 16 workers for up to 6 h, but the runtime was often considerably lower owing to early stopping. For both pre-training and fine-tuning, a worker consists of an Nvidia Volta graphics processing unit (GPU) and 10 central processing units (CPUs), each with 2 GB of RAM. For the zero-shot mode (applying a pre-trained policy to a new netlist with no fine-tuning), we can generate a placement in less than a second on a single GPU.”
This is remarkably efficient compared to traditional approaches requiring months of human expert time-
We show that our method can generate chip floorplans that are comparable or superior to human experts in under six hours whereas humans take months to produce acceptable floorplans for modern accelerators. Our method has been used in production to design the next generation of Google TPU.
Let's now look at it at a deeper level.
A Deeper Look at the AI behind AlphaChip
The AI is predicated on a simple intuition- if a supervised learning model can accurately assess the label that would be associated with a particular state (especially of unseen states), then it has built a solid internal representation of the state ( in other words, it has extracted good features with which it can model the state).
Introduction to Edge Graph Neural Networks
While both Edge-GNNs and standard GNNs operate on graph-structured data, their approaches to learning representations differ significantly. This difference is crucial for AlphaChip’s success in capturing the complex relationships between chip components.
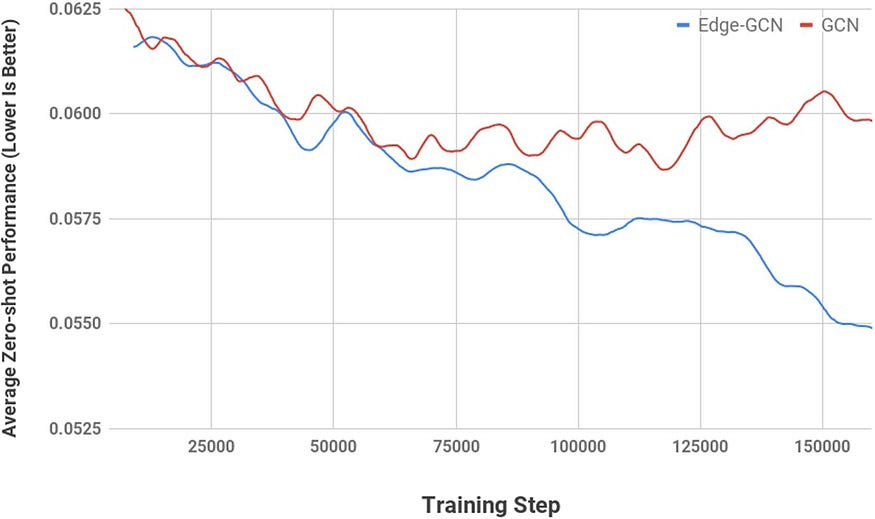
Let’s break down the training process and highlight the key distinctions:
Node Initialization: Each node is initialized with a feature vector representing its attributes.
Message Passing: Each node aggregates information from its neighboring nodes. This aggregation typically involves a function like summation, averaging, or max-pooling.
Node Update: The node’s representation is updated based on the aggregated information and its own previous representation.
Iterative Process: This message-passing and node-update process is repeated for several iterations, allowing information to propagate throughout the graph.
While standard GNNs implicitly consider edge information through the neighborhood structure, they don’t explicitly model edge features. This can be a limitation when the relationships between nodes are as important as the nodes themselves, as is the case in chip design. This is a key reason that standard GNNs may struggle to capture complex, multi-hop relationships between nodes
Node Initialization: Each node is initialized with a feature vector representing its attributes (type, width, height, etc.).
Edge Initialization: Each edge is initialized with a feature vector representing its attributes (e.g., number of connections, distance).
Edge Updates: Each edge updates its representation by applying a fully connected network (or another suitable function) to a concatenation of the representations of the two nodes it connects and its own previous representation. This allows the network to learn about the specific relationship between the connected components.
Node Updates: Each node updates its representation by taking the mean (or another aggregation function) of all incoming and outgoing edge representations. This allows the network to aggregate information about the node’s connections.
Iterative Process: These edge and node updates are performed iteratively, allowing information to propagate throughout the graph and learn complex relationships between components.
This is more important than you’d think.
Why Edge-GNNs create Better Representations than GNNs
The key innovation is in the update mechanism: edges first update their representations using concatenated node features and learned edge weights, then nodes update by aggregating their connected edge representations. This architectural choice is crucial because it enables learning patterns like “components with high data flow should be placed closer” directly from the connectivity structure, creating representations that generalize across different chip designs.
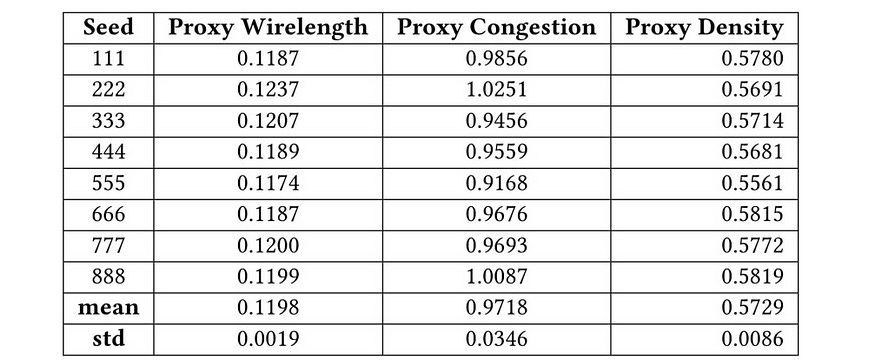
This supervised foundation makes the reinforcement learning more stable and efficient. I might be biased here, but in my mind, this continues to prove my working hypothesis that behind every successful implementation of RL is Supervised Learning doing most of the heavy lifting.
To reiterate, our SL protocol gives us an edge GNN that creates good representations of our state. These representations can now be fed into the RL-based planner- helping us actually create the chips.
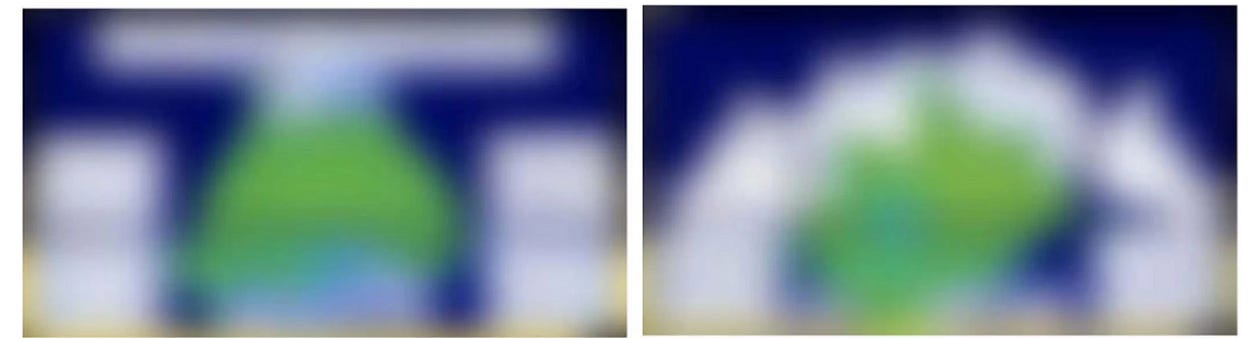
Using Reinforcement Learning for Chip Building
While the supervised pre-training provides a strong foundation, it doesn’t directly optimize for the sequential decision-making process of chip placement. That is why AlphaChip uses reinforcement learning to guide the policy and value networks, which will be used by the placement agent to build the chip in the best way possible.
Policy and Value Networks: The policy network takes the output of the pre-trained Edge-GNN as input and outputs a probability distribution over possible placement locations. The value network takes the same input and outputs an estimate of the expected reward for that state.
Proximal Policy Optimization (PPO): The policy and value networks are trained using PPO, a state-of-the-art RL algorithm that ensures stable and efficient learning. PPO fits the chip design problem perfectly because it handles challenging multi-objective rewards and enormous state spaces through conservative policy updates. By clipping the policy change magnitud, it prevents destructive updates when good placements are found — crucial since rewards only come after complete placement. PPO’s ability to collect experiences in parallel also enables efficient training, letting AlphaChip learn from multiple chip designs simultaneously, which is essential given the computational demands of placement evaluation. To quote the brilliant Cameron R. Wolfe, Ph.D. and his excellent coverage of PPO- “When PPO is compared to a variety of state-of-the-art RL algorithms on problems with continuous action spaces, we see that it tends to learn faster and outperforms prior techniques on nearly all tasks”
Sequential Placement: The agent places macros one at a time, using the policy network to guide its decisions and the value network to evaluate the quality of its actions.
Reward Feedback: The agent receives a reward signal at the end of each placement episode, which is used to update the policy and value networks.
Both of these (+ the hierarchical placement) combine to create our high-performing chip designer. Given the very promising economics of this field, I think there is a lot of business value to be captured in this space, and I’m excited to see how it plays out.
As mentioned this is an excerpt. If you want to understand AlphaChip in more detail, checkout our main deep-dive on it over here.
Thank you for being here and hope you have a wonderful day.
Dev <3
I put a lot of work into writing this newsletter. To do so, I rely on you for support. If a few more people choose to become paid subscribers, the Chocolate Milk Cult can continue to provide high-quality and accessible education and opportunities to anyone who needs it. If you think this mission is worth contributing to, please consider a premium subscription. You can do so for less than the cost of a Netflix Subscription (pay what you want here).
If you liked this article and wish to share it, please refer to the following guidelines.
That is it for this piece. I appreciate your time. As always, if you’re interested in working with me or checking out my other work, my links will be at the end of this email/post. And if you found value in this write-up, I would appreciate you sharing it with more people. It is word-of-mouth referrals like yours that help me grow.
Reach out to me
Use the links below to check out my other content, learn more about tutoring, reach out to me about projects, or just to say hi.
Small Snippets about Tech, AI and Machine Learning over here
AI Newsletter- https://artificialintelligencemadesimple.substack.com/
My grandma’s favorite Tech Newsletter- https://codinginterviewsmadesimple.substack.com/
My (imaginary) sister’s favorite MLOps Podcast (here)-
Check out my other articles on Medium. : https://rb.gy/zn1aiu
My YouTube: https://rb.gy/88iwdd
Reach out to me on LinkedIn. Let’s connect: https://rb.gy/m5ok2y
My Instagram: https://rb.gy/gmvuy9
My Twitter: https://twitter.com/Machine01776819