The Current State of AI Markets [Guest]
A Quantitative Look at where Value has accrued in the AI Value Chain
Hey, it’s Devansh 👋👋
Our chocolate milk cult has a lot of experts and prominent figures doing cool things. In the series Guests, I will invite these experts to come in and share their insights on various topics that they have studied/worked on. If you or someone you know has interesting ideas in Tech, AI, or any other fields, I would love to have you come on here and share your knowledge.
I put a lot of effort into creating work that is informative, useful, and independent from undue influence. If you’d like to support my writing, please consider becoming a paid subscriber to this newsletter. Doing so helps me put more effort into writing/research, reach more people, and supports my crippling chocolate milk addiction. Help me democratize the most important ideas in AI Research and Engineering to over 100K readers weekly. Many companies have a learning budget that you can expense this newsletter to. You can use the following for an email template to request reimbursement for your subscription.
PS- We follow a “pay what you can” model, which allows you to support within your means, and support my mission of providing high-quality technical education to everyone for less than the price of a cup of coffee. Check out this post for more details and to find a plan that works for you.
Eric Flaningam is my favorite resource for understanding the business side of the Tech Markets. His ability to look both across the industry and go deep into specific topics is unmatched. Every article I’ve read from him has given me a new perspective on the developments in the space.
This article will answer (in more detail than you’d think possible) a key question on many people’s mind- “Where is all the money in Gen AI?” I love this piece for 2 key reasons-
Eric's data points and analysis are very different from the kinds of information I would typically examine, which brings a fresh perspective to the newsletter (the whole reason I wanted to introduce guest posts to begin with). For example, his wonderful analysis of data centers and how they fit into long-term market shifts is not an angle I’d ever considered.
Eric’s approach to systematically breaking down the value of Gen AI into different components is a great template for us to start utilizing when doing estimates for the ROI of prospective projects.
Eric shares his insights through the Substack publication Generative Value. Would very strongly suggest signing up for it to never miss his insight ( you should also join my Eric Flaningam fanclub).
1. The AI ROI Debate
For the first time in a year and a half, common opinion is now shifting to the narrative “Hyperscaler spending is crazy. AI is a bubble.” Now, I’m not one for following public opinion, but investing is about finding undervalued opportunities; the more pessimism, the better.
We shouldn’t be surprised at the increasing pessimism around AI. The gap between infrastructure buildout and application value was expected. For every large infrastructure buildout, capacity never perfectly meets demand. And that’s okay. There will not be a perfect transition from infrastructure build-out to value creation.
In part, the recent rise in AI pessimism came from the latest hyperscaler capital expenditure numbers.
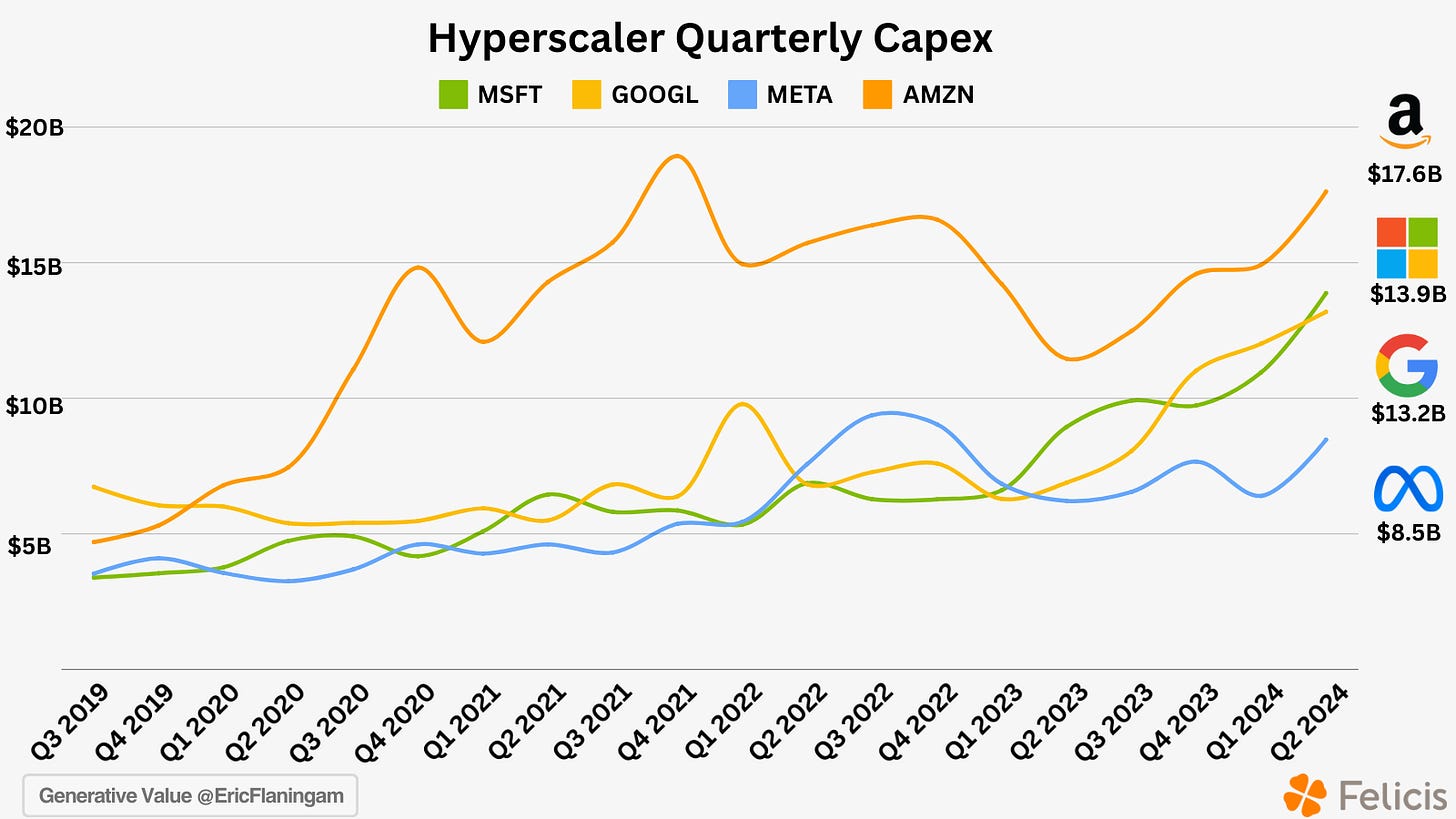
Amazon, Google, Microsoft, and Meta have spent a combined $177B on capital expenditures over the last four quarters. On average, that grew 59% from Q2 of 2023 to Q2 of 2024. Those numbers are eye-watering, but two things can be true:
There’s not a clear ROI on AI investments right now.
Hyperscalers are making the right CapEx business decisions.
We need to separate the conversation between ROI on AI investments and ROI on hyperscaler AI spending. ROI on AI will ultimately be driven by application value to end users. When a company decides to build out an AI app, it’s based on the potential for revenue or cost replacement.
For the large cloud service providers, there’s a different equation.
~50% of the spend is on the data center “kit” like GPUs, storage, and networking. Thus far, this has been demand-driven spending! All three hyperscalers noted they’re capacity-constrained on AI compute power.
Much of the other 50% is spent on securing natural resources that will continue to be scarce over the next 15 years: real estate and power.
They’ll do this themselves or through a developer like QTS, Vantage, or CyrusOne. They buy real estate for data centers with proximity to compute end usage, power availability, and cheap real estate. They’ve acquired most of the prime locations for data centers already; now, they’re buying locations primarily based on power availability. Power will continue to be a bottleneck for compute demand, and the hyperscalers know it.
The equation for hyperscalers is relatively simple: data centers are at least 15-year investments. They are betting compute demand will be higher in 15 years (not a crazy assumption).
If they do not secure these natural resources, three things could happen:
They will lose business to competitors who have capacity.
They’ll have to secure sub-optimal land with worse price/performance profiles.
Challengers will buy this land and power capacity and attempt to encroach on the hyperscalers.
If they secure this land and don’t need the computing power right away, then they’ll wait to build out the “kit” inside the data center until that demand is ready. They might’ve spent tens of billions of dollars a few years too early, and that’s not ideal. However, the three big cloud providers have a combined revenue run rate of $225B.
As Google’s CEO Sundar said, “The risk of under-investing is higher than the risk of over-investing.”
Thus far, we’ve started to see the buildout of AI infrastructure in the form of GPU purchases and data center construction costs, and we’re at the point where we ask ourselves, “Where has value been created thus far, and where will it be created moving forward?”
2. A Summary of the Current State of AI Markets
Currently, most AI value has accrued to the infrastructure level, specifically Nvidia and the companies exposed to it. We’re starting to see the buildout of “AI Data Centers”, but we’re early on in this trend, and energy is a real bottleneck to this buildout.
The cloud providers are seeing some revenue from model APIs or “GPUs as a Service”, where they buy GPUs and rent them out via the cloud.
We haven’t seen wide-scale application revenue yet. AI applications have generated a very rough estimate of $20B in revenue with multiples higher than that in value creation so far (cost reduction), but this pales in comparison to infrastructure costs.
The industry will ultimately be driven by AI application value (either revenue or cost replacement). That value will be driven by the end value to the consumer or enterprise.
So, we come to this conclusion: the bigger problems that AI applications can solve, the more value will accrue across the value chain.
At this point in time, we’ve seen a massive amount of infrastructure investment with the expectation of application revenue. The question is this: where is the meaningful value creation from AI?
The answer is that it’s unclear. It’s early. And that’s okay.
Before we dive in, I want to map out a theoretical framework for how I think about AI investing (of course, this is simplified).
Broadly, we have semiconductors (and their value chain), data centers (and energy), cloud platforms, models, data, and the application layer.
3. AI & Semiconductor Markets
Summary: Nvidia is taking the lion’s share of AI semiconductor revenue, Nvidia’s supply chain has benefitted to varying extents, and HBM memory & exposure to custom ASICs follow those two categories.
Nvidia, Nvidia’s Supply Chain, and AMD
Last quarter, Nvidia did $26.3B in data center revenue, with $3.7B of that coming from networking. Tying back to the Capex numbers from earlier, 45% of revenue or $11.8B came from the cloud providers.
TSMC, the semiconductor foundry manufacturing Nvidia’s leading-edge chips, is the most direct beneficiary of Nvidia’s AI revenue, commenting:
“We forecast the revenue contribution from several AI processors to more than double this year and account for low-teens percent of our total revenue in 2024. For the next five years, we forecast it to grow at 50% CAGR and increase to higher than 20% of our revenue by 2028. Several AI processors are narrowly defined as GPUs, AI accelerators and CPU's performing, training, and inference functions and do not include the networking edge or on-device AI.”
TSMC’s average 2024 revenue estimate is $86.3B; estimating 12% of that from AI gets us to a projected AI revenue of ~$10.4B.
As TSMC’s revenue grows, eventually, so does their CapEx, generating revenue for the semiconductor capital equipment providers as well.
And what about AMD?
In last quarter’s earnings, Lisa Su said she expected MI300X revenues to surpass $4.5B, up from the previously expected $4B. But, the most important note came in the earnings call prior where she commented:
“From a full year standpoint, our $4 billion number is not supply capped.”
This is not an ideal position to be in when your biggest competitor is selling ~$20B of GPUs and can’t sell enough. With that being said, it takes time to develop chip lines, and going from chip release to $4B+ in revenue in a year is an impressive achievement.
HBM, Broadcom, and Marvell
High-bandwidth memory chips are designed to reduce the memory bottleneck for AI workloads; SK Hynix, Samsung, and Micron are the three manufacturers of these chips.
According to Trendforce, “Overall, SK Hynix currently commands about 50% of the HBM market, having largely split the market with Samsung over the last couple of years. Given that share, and DRAM industry revenue is expected to increase to $84.150 billion in 2024, SK Hynix could earn as much as $8.45 billion on HBM in 2024 if TrendForce's estimates prove accurate.”
Additionally, Marvell and Broadcom provide two primary AI products:
The semiconductors for most networking equipment in data centers (supplying customers like Arista and Cisco).
Codesign partnerships helping companies build custom AI chips (the best example is Broadcom and Google’s partnership for developing TPUs).
Broadcom: “Revenue from our AI products was a record $3.1 billion during the quarter” driven by the TPU partnership with Google and networking semiconductors. Last quarter, they disclosed about ⅔ of that comes from TPUs and ⅓ from networking.
Marvell: “We had set targets of $1.5 billion for this year and $2.5 billion for next year…all I can tell you is both of them have upsized.”
Summarizing the semiconductor section, we see Nvidia at an $105B AI run rate, Broadcom at a $12.4B run rate, AMD with an expected $4.5B in 2024 AI revenue, TSMC with an expected $10.4B in AI revenue, the memory providers earning ~$16B in HBM revenue (SK Hynix with ~46-52% market share, Samsung with ~40-45% market share, Micron with ~4-6% market share), and Marvell likely around ~$2B in AI revenue.
4. AI Data Center Markets
The AI Data Center narrative is interesting. We’re seeing revenue flow to two locations: GPUs/AI Accelerators and data center construction. We haven’t seen revenue flow to traditional data center equipment providers like Arista, Pure Storage, or NetApp.
Data Center Expenditures
The most direct driver of data center construction is hyperscaler capital expenditures. Amazon, Google, Microsoft, and Meta have spent a combined $177B on CapEx over the last four quarters. On average, that grew 59% from Q2 of 2023 to Q2 of 2024.
Approximately 50% of that goes directly to real estate, leasing, and construction costs.
Synergy Research notes that it has taken just four years for the total capacity of all hyperscaler data centers to double, and that capacity will double again in the next four years.
"We're also seeing something of a bifurcation in data center scale. While the core data centers are getting ever bigger, there is also an increasing number or relatively smaller data centers being deployed in order to push infrastructure nearer to customers. Putting it all together though, all major growth trend lines are heading sharply up and to the right."
It’s clear data centers are being built, but who benefits from this revenue?
Theoretically, value should flow through the traditional data center value chain:
The interesting divergence in this data is that we haven’t seen revenue flow to traditional data center networking and storage providers…yet. That means revenue thus far is mostly going to rack-scale implementations and data center construction.
Data center developers like Vantage, QTS, and CyrusOne buy land and power, who then lease that out to big tech companies. The developers will then go through the construction process to build the data center before it’s filled with equipment.
The other primary beneficiaries thus far have been server manufacturers like Dell and SMCI, who deliver racks with Nvidia GPUs. Dell sold $3.2B worth of AI servers last quarter, up 23% Q/Q. SMCI’s revenue last quarter was $5.3B, up 143% Y/Y.
What About Energy?
It’s clear there will be value created for the energy value chain; I’ll be publishing more on this in future articles. Demand is increasing, and the question is what bottlenecks will be alleviated to fulfill that demand (I don’t have a good answer to that question right now).
One anecdote to highlight the ensuing energy demand:
PJM Interconnection, the largest electrical grid operator in the US, had its annual energy auction the week of July 22nd, 2024. PJM services the northern Virginia region, which is the largest data center hub in the world. Their auction ultimately sold $269.93/MW-day for most of their region, up from $28.92/MW-day for their last auction.
Prices approximately 10xed from this year to next. This highlights the energy concern for the hyperscalers, and how creative they’re willing to get to secure capacity.
5. AI Cloud Markets
I covered this more fully in my quarterly cloud update here. All three hyperscalers want to be vertically integrated AI providers, allowing customers to tap into whichever service level they prefer (infrastructure, platforms, applications). This happens to be the exact playbook they’ve been successfully deploying with traditional cloud software.
Microsoft has undoubtedly executed the best thus far and gives us a good idea of cloud AI revenue. We can do some back-of-the-napkin math to estimate their Azure AI revenue. For each of the last 5 quarters, they’ve disclosed Azure AI revenue growth contribution. Combining that with net new Azure revenue gives us an idea of how much AI revenue they added each quarter:
They hit approximately a $5B Azure AI run rate last quarter.
Google and Amazon both noted they have “billions” in AI revenue run rate but didn’t disclose details past that.
Oracle has signed $17B worth of AI compute contracts (this is RPO, so it will be recognized over a 3-5 year period, depending on the specific contract. If we average that out over 12 quarters, Oracle will be doing around $1.3B in AI revenue a quarter, which puts it in the same vicinity as the other hyperscalers.
Let’s throw in the GPU Cloud data to round out the picture for AI revenue:
Coreweave did an estimated $500M in revenue in 2023, and is contracted for $2B in revenue in 2024. Lambda Labs did an estimated $250M in 2023 revenue. Crusoe is estimated to have done $300M+ in 2023 revenue. Combined these companies will add a few billion more to the AI Cloud pie.
Summarizing this section: we have annual run rates of a very rough estimate of ~$20B thus far for AI cloud services.
Everything discussed above is what I consider “AI infrastructure”, and has been built out to support the eventual coming of AI application value. If I can point out the STEEP drop off in revenue from semiconductors, to data centers, to the cloud. This tells the story of AI revenue so far.
Semiconductors will earn $100-$200B in AI revenue this year, hyperscalers have spent $175B+ in capex over the last four quarters, the cloud providers will do ~$20-25B in AI revenue this year.
The cloud revenue gives us the real indication of how much value is being invested into AI applications; needless to say it’s much less than the infrastructure investments thus far.
6. AI Models & Application Revenue
AI application value is the most important question in AI markets right now. AI application value will ultimately drive investments across the value chain. All of this infrastructure buildout becomes a bubble without value creation on the backend.
The scale of AI application revenue will be determined by the value it creates for customers. What are the problems it solves? What’s the scale of those problems?
Quite frankly, we don’t have good answers to that right now. Sapphire estimated some of these figures below:
Data companies like Scale AI (reportedly at $1B in revenue this year) and VAST Data ($200M in ARR last year, much higher this year) show the clear demand for AI apps. However, they still fall into an infrastructure-like category, not directly touching the end user.
Our best indication of AI app revenue comes from model revenue (OpenAI at an estimated $1.5B in API revenue, Anthropic at an estimated 2024 $600M revenue). Freda Duan from Altimeter broke down the projected financials for OpenAI and Anthropic.
The problem comes with analyzing ROI on this revenue. For LLM-based applications, we could assume an 80% gross margin and say between $5B-$10B in application revenue has been sold. However, what about the value created from labor replacement costs like Klarna saving $40M on customer service costs?
I can confidently say two things:
AI application will not generate a net positive ROI on infrastructure buildout for some time.
AI applications must continue to improve and solve new problems to justify the infrastructure buildout.
On the latter point, it does appear that the future of AI is agentic (LLMs with memory, planning, and tool integrations to execute tasks). This seems to represent the next potential step function of AI application value. In reality, AI value may manifest itself in ways we can’t predict. (How could you predict in 2000 the internet would give rise to staying in other people’s houses, or getting rides in other people’s cars?)
As Doug O’Laughlin pointed out, we’re in the very early stages of AI:
New technologies are hard to figure out, especially in the short term. And that’s okay. Value will be created in unforeseen ways. Over the long run, betting against technology is a bad bet to make; and certainly won’t be one I’m making.
I provide various consulting and advisory services. If you‘d like to explore how we can work together, reach out to me through any of my socials over here or reply to this email.
I put a lot of work into writing this newsletter. To do so, I rely on you for support. If a few more people choose to become paid subscribers, the Chocolate Milk Cult can continue to provide high-quality and accessible education and opportunities to anyone who needs it. If you think this mission is worth contributing to, please consider a premium subscription. You can do so for less than the cost of a Netflix Subscription.
If you liked this article and wish to share it, please refer to the following guidelines.
That is it for this piece. I appreciate your time. As always, if you’re interested in working with me or checking out my other work, my links will be at the end of this email/post. And if you found value in this write-up, I would appreciate you sharing it with more people. It is word-of-mouth referrals like yours that help me grow. . You can share your testimonials over here.
Reach out to me
Use the links below to check out my other content, learn more about tutoring, reach out to me about projects, or just to say hi.
Small Snippets about Tech, AI and Machine Learning over here
AI Newsletter- https://artificialintelligencemadesimple.substack.com/
My grandma’s favorite Tech Newsletter- https://codinginterviewsmadesimple.substack.com/
Check out my other articles on Medium. : https://rb.gy/zn1aiu
My YouTube: https://rb.gy/88iwdd
Reach out to me on LinkedIn. Let’s connect: https://rb.gy/m5ok2y
My Instagram: https://rb.gy/gmvuy9
My Twitter: https://twitter.com/Machine01776819